Reliability and validity in measurement are important in the research process.
What is Measurement?
Measurement is at the heart of any scientific discipline. The primary function of measurement is to assign numbers to objects or events according to certain rules. It is the process by which empirical data are organized in some systematic relationship to the concept being studied.
Bryman identifies three main reasons for the preoccupation with measurement in quantitative research:
- Measurement allows us to delineate fine differences between people regarding the characteristics in question.
- Measurement gives us a consistent device or yardstick for making such distinctions.
- Measurement provides the basis for more precise estimates of the degree of relationship between concepts (e.g., through correlation analysis).
Measurement theory contends that, however precise our instruments for measuring and however careful our efforts of observations are, there will always be some errors introduced into our measurement. This is what we refer to as measurement error.
The central formulation of measurement theory states that an observed measure (score) is equal to the true score plus some error (above or below the true score), which necessarily occurs in the process of observing the phenomenon.
Thus if X is an observed score, T a true score and e a measurement error, then the relationship between X, T, and e for the i-th individual can be expressed as:

Thus for specified measurement i, the measurement error e1 is the difference between X, and 7):

Whenever there is a measurement of an attribute, three kinds of error are likely to be generated in the measurement process. These are (i) random error, (b) systematic error, and (c) situational error.
A random error appears as an unexplained variation. It occurs randomly. A random error exhibits no systematic tendency to be either negative or positive.
If the errors are truly random, they will cancel each other, and the overall average of these errors will be zero.
That is;
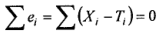
Sometimes people do not know their exact age. While reporting, some people will overstate their age, and some will understate. This will produce random errors.
The inaccuracy of measurements that repeatedly occurs and for the same reasons is labeled systematic error.
Observations consistently overestimate or underestimate the true value are subject to systematic error. Systematic errors frequently occur as a result of improper research procedures such as;
- Faulty conceptualization of the research problem.
- A poorly designed research instrument.
- The researcher’s bias. and
- Incorrect operationalization of variables.
Unlike random error, the sum of the systematic errors does not add to zero.
In the measurement of contraceptive use, for example, if people consistently report higher use, because they believe the interviewer or the program managers would appreciate this, errors would take place systematically, giving a false high rate of contraceptive use.
Likewise, a defective weighing machine or any other defective measuring device is likely to produce a systematic error in the measuring process.
Situational errors are ones that occur neither at random nor systematically but occur as a result of particular personal characteristics of the individual(s) or other special circumstances that can influence the measurement process.
Fatigue, for example, of the respondents or an unusual environmental detraction, may generate a situational error.
Social researchers primarily employ two criteria for evaluating the quality of their measures.
These are;
- reliability, and
- validity.
These two criteria seek to answer;
- how consistent or dependable (which refers to reliability), and
- accurate (which refers to validity) is the information obtained?
We discuss these concepts in turn in the following sections.
Reliability and its Measurement
Put simply, reliability is the degree to which a measuring procedure produces similar, in other words, consistent outcomes when it is repeated under similar conditions.
A tailor measuring fabric with a measuring tape obtains a value of the length of the fabric as 20 inches.
If the tailor takes repeated measures of the fabric and each time comes up with the same length, it is said that the tape measure is reliable.
The essential conditions in such a measurement process are that repeated administration of the same instrument must be made under essentially similar conditions, and the object will not be changed over time.
Reliable instruments are robust; they work well at different times under different conditions.
However, if the object being measured does change in value, the reliability measure will indicate that change.
How can a measuring instrument be unreliable?
If your weight remains constant at 60 kilograms, but repeated measurements on your bathroom scale show your weight to fluctuate, the lack of reliability may be due to a weak spring inside the scale.
In the case of social research, unreliability in the questionnaire as a measuring instrument might arise in the measurement of any concept due to such things as a question or answer categories being so ambiguous that the respondent is unsure how he or she should answer.
In the given circumstances, the respondent fails to answer consistently. This leads to the unreliability of the measuring instrument (here the questionnaire).
The credibility and testability of any theory thus depend primarily upon adequate measurement tools or devices in social research.
The reliability of a measure can be described in terms of three interrelated concepts:
- Stability;
- Equivalence; and
- Internal consistency.
A measure is said to be stable if you can secure consistent results with repeated measurements of the same individuals or objects with the same instruments.
An observational procedure or measuring device is stable if it gives the same reading on an individual or object when repeated one or more times.
The second perspective on reliability, i.e., equivalence, considers how much error may be introduced by different investigators (in observation) or different samples of items being studied (in questioning or scales).
Thus, while stability is concerned with personal and situational fluctuations from one time to another, equivalence is concerned with variations at one point in time among observers and samples of items.
A good way to assess the equivalence of measurement by different observers is to compare their scoring or evaluation of the same event.
In the 1993 IDD prevalence survey in Bangladesh, for example, eight physicians measured the goiter grade of a single person at a single point in time to assess the extent of error incurred in the measurement due to the physicians.
The third approach to reliability, i.e., the internal consistency, uses only one administration of an instrument or test to assess the consistency or homogeneity among the items.
It must always be kept in mind that reliability refers to a specific measuring instrument applied to a specific population under a specific condition.
To assess the reliability of any test based on at least interval data, we can use, in general, Pearson’s product-moment correlation coefficient r, and the resulting correlation will be our estimate of the reliability coefficient.
The coefficient varies between ‘0’ (no correlation and, therefore, no internal consistency) to ‘1’ (perfect correlation and, therefore, complete internal consistency).
It is usually expected that a coefficient of 0.8 and above implies an acceptable level of reliability.
The correlation must be statistically significant to establish reliability, and the strength of the correlation provides information regarding the dependability of the measures involved.
In estimating reliability, one can make use of different sources of variation in measurement values.
The simplest approach is the so-called variance approach, which makes use of the preceding equation [ (a), viz. Xt = Ti+ei. ]
The set of the observed scores has a total variance Vo (say). Likewise, the true scores (Tj) and the errors of measurement e will have variances Ft and Ve, respectively.
The total variance is the sum of the true variance and the error variance, that is;

The ratio of the true variance to the total variance is the reliability coefficient. That is;
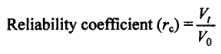
Since Vt is less than or equal to Ko, the reliability coefficient can take on a maximum value of 1.
When this is so, we say that the observed scores are the same as the true sores, and in that instance, perfect reliability has been assumed to be achieved.
An alternative formula for the reliability coefficient can be obtained by dividing both sides of the expression (d) by Vq.
Thus;
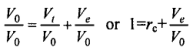
After some rearrangements;

There are several other popular techniques available for measuring reliability. These are
- Test-retest method
- Parallel-forms method
- Split-half method,
- Kuder-Richardson formula and
- ANOVA
The last three techniques are employed to assess the internal consistency aspect of reliability.
How to Increase Reliability
The following techniques can increase the estimated reliability of a test:
- Increase the length of a test by adding more items. The longer the test is, the higher the likely reliability. One should only add items that have been selected from the same population of items.
- You can achieve enhanced reliability through improved investigator consistency by using only well-trained, supervised, and motivated persons to conduct the research.
- Increase the variance by making the test more discriminating. Add items that separate persons along a continuum, with some items that only the top scores will get right, some only the worst scores will get wrong, and so on up and down the line. Please get rid of items that everybody gets right (as well as those that everyone gets wrong), since they are not providing any power to discriminate. A 2-item test obviously cannot yield as much variation as a 100-item test, nor will the 2-item test provide much information on the discriminating power of the items other than, at best, perhaps sorting the above-average from the below-average.
- Increase the size of the sample being tested. No matter how many items you have or how well your items discriminate, if there are not enough subjects of varying ranges of ability, the reliability of the test can be severely underestimated.
Validity and its Measurement
For the test to have any practical use, it must not only be reliable, but it also must be valid. A traditional definition of the validity of a measuring device is the extent to which it measures what it was designed to measure.
That is, if a test has been designed to measure musical aptitude, for example, a valid test measures just that, and not some other extraneous variable(s).
When you step on the scales, you want to know your weight, not your IQ or some other unknown quality.
In scientific usage, a measurement of a given phenomenon (as designated by a given concept) is viewed as a valid measure if it successfully measures the phenomenon.
It seems clear that the definition of validity has two parts;
- that the measuring device is measuring the concept in question and not some other concepts;
- that the concept is being measured accurately.
One can have the first without the second but not vice-versa (the concept cannot be measured accurately if some other concept is being measured).
To clarify the concept more clearly, assume that we wish to measure IQ and that Afra certainly has some actual level of IQ (say 110).
We can construct a test that measures her IQ but measures it with some degree of error.
That is, it measures her intelligence as 100 when it is 110.
No doubt, such a test is measuring the right concept (IQ) and has some potential for being a valid measurement if we can refine it to remove the error in measurement.
On the other hand, if we wish to measure Afra’s intelligence, we may devise a test that does not measure intelligence at all.
Such a test can never be a valid measure of intelligence as it is measuring some other concept (such as her skill, or maybe measuring nothing at all), rather than measuring intelligence as we had planned.
The difficulty is that this test may still yield a numerical score, and could conceivably even yield a score of 110 for Afra.
However, it still is not valid because it does not measure the desired phenomenon.
Thus, it becomes very important to be able to assess the validity of tests, as all of them will yield some sort of score, but this does not necessarily mean that the measurement is valid.
By definition, if a measure is valid, it will be accurate every time, and thus be reliable also, but the converse is not true. That is a reliable measure that may not be valid.
Another way of putting the same statement is that reliability is a necessary condition but not a sufficient condition for validity.
The validity of measurement can be viewed broadly in two ways: internal validity and external validity.
Internal validity seeks to answer whether a difference exists at all in any given comparison. It asks whether or not an apparent difference can be attributed to the same measurement artifact.
If one does not encounter contradictions in the data within a given experiment, then the study is said to have internal validity. Contradictory findings signal the absence of internal validity.
External validity is the problem of interpreting the difference, the problem of generalization of the experimental research.
Even if internal validity is present, the findings are not said to have external validity unless they are held to be valid for additional (external) situations besides the original study that generated the findings.
Many forms of validity are mentioned in the research literature, and the number grows as we expand the concern for more scientific measurement.
One accepted classification consists of four major forms;
- face validity.
- content validity,
- criterion-related validity, and
- construct validity.
We discuss these in brief.
Face Validity
The most basic testing method for validity is to carefully examine the measure of a concept in the light of its meaning and to ask oneself seriously whether the measuring device seems to be measuring the underlying concept. This form of careful consideration and examination is referred to as face validity.
Face validity is based on the test’s general appearance; as such, a test will have more face validity if its look and feel are consistent with the construct under study.
From a testing perspective, it is really important to have a test that looks on the surface like it can tap the characteristic under study.
This surface appearance of the test may motivate the test-takers, especially when the test produces an assumption of relevance.
For example, a test for measuring a person’s aptitude for banking might be more realistic and motivating if it asks questions about balance sheets and interest payments, rather than, say, the batting average of a certain cricket player.
What is important invalidity context is that a test has face validity if the items are reasonably related to the perceived purpose of the test.
Face validity reflects the content in question.
It is simply assessed by asking other people whether the measure seems to be getting at the concept that is the focus of attention.
In other words, people, possibly those with experience or expertise in a field, might be asked to act as judges to determine whether on the face of it the measure seems to reflect the concept concerned.
Face validity, thus is essentially an intuitive process that judges whether the measuring instrument arrives at the concept adequately.
Content Validity
Content validity seeks to answer whether the empirical indicators (tests, scales, questions, etc.) fully represent the domain of the underlying concept being studied.
In this sense, content validity concerns the degree to which the content of a set of items adequately represents the universe or domain of all relevant items under study.
To the degree that the items reflect the full domain of content, they can be said to be content-valid.
The determination of content validity is judgmental and can be approached in several ways. Two of the approaches are:
- The designer may determine it by carefully defining the topic of concern, the items to be scaled, and the scales to be used. This logical process is often intuitive and unique to each research designer.
- One may use a panel of persons to judge how well the instrument meets the standards.
The major problems with content validity arise when
- There is no consensus about the definition of the concepts to be measured.
- The concept is a multi-dimensional one consisting of several subconcepts
- The measure is lengthy and complex.
Criterion-related Validity
Criterion validity, variously called pragmatic validity, concurrent validity, or predictive validity, involves multiple measurements of the same concept.
The term concurrent validity has been used to describe a measure that is valid for measuring a particular phenomenon at the same time that the measurement is administered. In contrast, predictive validity relates to future performance on the criterion. These two measures thus differ only in a time perspective.
Predictive validity is concerned with how well a scale or instrument can forecast a future criterion and concurrent validity with how well it can describe a present one.
Look at the following cases:
- An opinion questionnaire is designed to forecast the outcomes of a bank employees’ union election. Our concern is to assess how correctly the questionnaire forecasts the results of the election. The more the questionnaire accurately forecasts the results, the more predictive validity the questionnaire possesses.
- An observational study is conducted to classify families as having a low, medium, and high income. The degree, to which the study can correctly classify these families, represents the concurrent validity.
- A questionnaire is designed to identify psychiatrically disturbed persons. The validity of the questionnaire might be determined by comparing its diagnoses with those made by a psychiatrist based on clinical investigation. The two approaches respectively lead to predictive validity and concurrent validity.
The opinion, income, and psychiatric conditions in the above examples are the criteria that we employ to measure the validity of the measuring instruments.
Ratings of performance, units produced in a certain period, amount of sales, and the number of errors made in a typing document are a few more examples of criteria.
Once the test specification is made and criterion scores are obtained, they may be compared. The usual approach is to correlate them using product-moment correlation.
For example, we might correlate test scores of a group of college students and the grade point average that these students made during their first year of college and regard this coefficient as a validity coefficient.
The validity coefficients usually tend to be much lower than the reliability coefficient (in the range .40-.60 with a median value of .50),
Construct Validity
Criterion-related validity is based upon empirical evidence (such as scores, income, and ratings) to serve as the basis for judging that what is being measured measures what it is supposed to measure (predictive capacity).
This is an empirically based form of validity, in which some observable evidence can be used to confirm the validity of a measure.
However, when there is neither a criterion nor an accepted universe of content that defines the quality being measured, then criterion-related validity cannot be used to test for validity.
The difficulty in applying rigorous and objective tests of validity in attitude measurement, for example, arises from the fact that such measurement is invariably indirect in the sense that the attitude is inferred from the verbal statements or responses.
An attitude is an abstraction, and it is generally impossible to asses its validity directly. An approach for this situation is that of construct validity.
Attitude, aptitude, and personality tests generally concern concepts in this category.
Construct validity is based on forming hypotheses about the concepts that are being measured and then on testing these hypotheses and correlating the results with the initial measure.
Here is a 3-step procedure to test the construct validity of measures:
- Specify first the theoretical relationship between the concepts themselves.
- Examine the empirical relationship between the measures of the concepts.
- Interpret the empirical evidence in terms of how it clarifies the construct validity of the particular measure.
Suppose we develop two indices for measuring social class and label these indices as index-1 and index-2.
Assume that we have a theory that contains a hypothesis which states that an inverse relationship exists between social class and crime-as social class increases, crime decreases.
Assume that this hypothesis has been tested by measuring social class by index-1 and has been established by statistical tests.
Construct validity consists of replacing index-1 by index-2 in theory and retesting the entire theory. If we get the same results for the whole theory (especially for the hypothesis containing index-2) as when we used index 1 to measure social class, then we say that the new measure (index-2) has construct validity.
The types of validations discussed so far can be seen as an accumulation, with each of the subsequent types of validation, including all of the elements of all former types, along with some new features.
In other words, construct validation requires more information than criterion validation, and criterion validation requires more information than content or face validation.
For this reason, construct validation is often said to be the strongest validation procedure. In summarizing the various types of validity testing for their effectiveness in social research, Zeller and Carmines conclude that construct validity is the most useful and applicable to the social sciences.
To conclude, it must be noted that the reliability and validity of measurement are not invariant characteristics. They are always specific to a particular population, time, and purpose.
In any given situation, the researcher, therefore, has to decide what degree of unreliability and invalidity he will consider as acceptable.
Analysis of Variance in Estimating Reliability
The well-known analysis of variance techniques can be applied to assess the internal consistency test reliability. This technique can be employed for establishing rtt one for the situation in which the items are dichotomously scored (right-wrong, agree-disagree), and one in which the items are scored on an interval scale. We illustrate the method only with the dichotomous data.
The method presented here is due to Cyril Hoyt, a great statistician, and is popularly known as Cyril Hoyt method. We illustrate this method by example.
The following steps are involved in the computation of the reliability coefficient.
Example:-
A specialist has created a four-item test of shyness and given it to a random sample of five subjects. The test is scored only based on whether the item indicates shyness or whether it does not. It was scored as 1 for shyness and 0 for no shyness (see data below). Using the ANOVA technique, estimate the internal consistency reliability of the test.
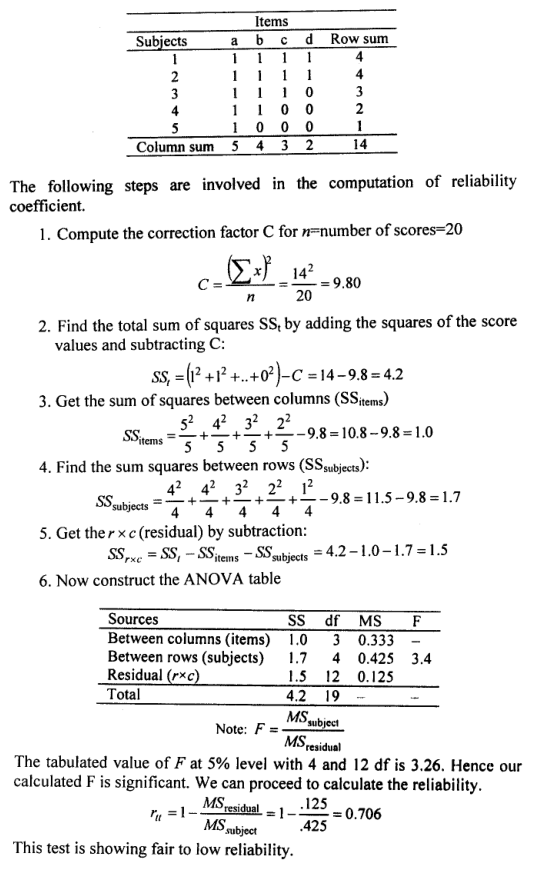
Constructing Measurement Scales
Scales are central to the subject of measurement in social research. A scale refers to an item or set of items (indicants) for measuring some characteristic or property, such as an attitude.
Scaling is a procedure for assigning numbers to a property of objects to impart some of the characteristics of numbers to the properties in question.
Thus, you may devise a scale to measure the paint’s durability (property).
In another context, you may judge a person’s supervisory capacity (property) by asking a peer group to rate that person on various questions (indicants) you create.
You may also measure the IQ score (property) of a person asking him pre-designed questions (indicants).
A scale generally represents a single complex concept, or construct, that combines multiple indicators into a common composite measure.
The reason scales are widely used in the social sciences is that many of the concepts social researchers want to study cannot be measured with a single indicator.
Concepts like religiosity are too multidimensional to be measured with a single item.
When such concepts are of interest, a scale or an index can be developed to try to incorporate the many facets of the abstract variable into a set of indicators representing its operational definition.
Scales are of two types: rating or ranking. Rating scales have multiple response categories and are also known as category scales.
They are used when respondents score an object or attitude without directly comparing it to another object or attitude.
For example, the respondents may be asked to rate the styling of a new shoe or quality of a garment on a five-point scale: excellent, good, average, below average, and poor, or frequency of visits of a community health worker: always, often, occasionally, rarely and never.
Ranking scales make comparisons among two or more attitudes or objects. The respondents under this method compare two or more objects and choose among them.
For example, respondents can express their attitudes by making a choice between two brands of car.
In such scaling, one may also follow the rank order. Under this approach, the respondents are asked to rank their choices according to the importance of the objects.
Scales can again be unidimensional or multidimensional. With a unidimensional scaling, one attempts to measure one attribute of the respondent or object.
One measure of employee potential is promotability. The manager may decide to identify the employee’s technical performance for promotion. It is a single dimension.
Several items may be employed to measure this dimension, and by combining them into a single measure, a manager may place employees along a linear continuum of promotability.
Multidimensional scaling recognizes that an object may be better described in an attribute of n dimensions rather than on a unidimensional continuum.
The employee promotability variable might be better expressed by three distinct dimensions: managerial performance, technical performance, and teamwork.
You Need To Know Reliability and Validity in Measurement.
What is the primary function of measurement in any scientific discipline?
The primary function of measurement is to assign numbers to objects or events according to certain rules. It organizes empirical data in a systematic relationship to the concept being studied.
What are the three main reasons for the preoccupation with measurement in quantitative research as identified by Bryman?
The three main reasons are:
- Measurement allows us to delineate fine differences between people regarding the characteristics in question.
- Measurement provides a consistent device or yardstick for making distinctions.
- Measurement offers the basis for more precise estimates of the relationship between concepts, such as through correlation analysis.
What is measurement error and how is it expressed in relation to observed scores and true scores?
Measurement error refers to the errors introduced into our measurement. The central formulation of measurement theory states that an observed measure (score) is equal to the true score plus some error, which occurs during the observation process. It’s expressed as: X (observed score) = T (true score) + e (measurement error).
What are the three types of errors likely to be generated in the measurement process?
The three types of errors are:
- Random error: Appears as an unexplained variation and occurs randomly.
- Systematic error: Consistently overestimates or underestimates the true value due to factors like faulty research procedures.
- Situational error: Occurs due to particular personal characteristics or special circumstances that influence the measurement process.
How is reliability defined in the context of measurement?
Reliability is the degree to which a measuring procedure produces similar or consistent outcomes when it is repeated under similar conditions.
What are the four major forms of validity testing?
The four major forms of validity testing are:
- Face validity,
- Content validity,
- Criterion-related validity,
- Construct validity.
What is the difference between internal validity and external validity?
Internal validity seeks to determine whether a difference exists in any given comparison and if the observed difference can be attributed to the measurement artifact. External validity concerns the generalization of the research findings, determining if they are valid for additional situations beyond the original study.